 |
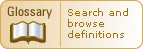
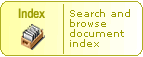
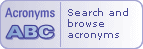
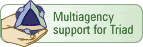
Triad is a Federal/State Interagency Partnership
|
 |
Adaptive Data Collection Strategies
A discussion of adaptive location selection and adaptive analytics selection as forms of adaptive data collection strategies.
The Triad approach and dynamic work strategies are most commonly used in the form of adaptive data collection strategies. Data collection strategies can be "adaptive" in a number of different ways, one or all of which may be used in a particular adaptive data collection program. These include:
- Adaptive Location Selection. Adaptive location selection refers to data collection programs where sampling location decisions are made in the field in response to real-time data collection results.
- One example is selecting biased locations in response to other information available once field work begins. The purpose usually is to confirm the presence or absence of contamination at levels of concern for a particular location. Biasing information can include visual inspection, non-intrusive geophysical survey results for subsurface sample selection, active or passive soil gas monitoring results, results from gamma walkover surveys in the case of radionuclide contamination, etc. Another common application is the use of direct push technologies in conjunction with real-time measurements to decide where to place more costly permanent monitoring wells, or to support evaluation of subsurface site hydrogeology.
- A second example is the selection of additional locations in the field in response to contamination that has been discovered. The purpose is usually to laterally or vertically bound contamination above some pre-defined concentration level (i.e., contaminant delineation), or to pursue the footprint of contamination migration that is taking place (e.g., a groundwater contaminant plume). This type of application is particularly well-suited to geostatistical techniques, since the concept of spatial autocorrelation and its effective range is critical to selecting appropriate spacing between samples.
- A third example is the selection of an area (or definition of a decision unit) for the application of systematic sampling based on real-time results. During a remedial investigation, this approach can be used to more cost-effectively satisfy risk-assessment data needs. During remediation, this strategy is particularly effective for supporting closure decisions for particular decision units. The first decision to be made based on real-time results is whether an area needs remediation, or is ready for closure. If the latter, the second decision is the level of closure sampling required (i.e., the number of samples and so grid spacing). For statistically-based closure programs, the number of samples required is usually a function of the average value of residual contamination expected and the variability one expects to see in contamination levels across a decision unit. Real-time results can provide information about both, and so support a systematic sampling strategy customized for a particular decision unit. This assumes that the analytical uncertainty associated with real-time results is small compared to the spatial variability of contamination levels in a particular area, which is usually the case.
- Adaptive Analytics Selection. Adaptive analytics selection refers to data collection programs where sample analysis decisions are made in the field in response to real-time measurement results.
- One example is selecting a sample for more definitive analysis based on a real-time measurement result. This is a common adaptive strategy when real-time measurements yield results in the region of decision uncertainty, requiring additional analysis to obtain a more definitive result. For radionuclides, one might submit a sample for gamma spectroscopy analysis when a gamma walkover survey indicated an anomaly. For PCBs, one might submit a sample for GC analysis when a test kit result identified a sample as potentially containing PCBs. For volatile organics, one might require GC/MS analysis when a PID or FID headspace analysis on a soil sample indicated the presence of elevated volatile organic content.
- A second example is when sampling methods (preparative and/or determinative) are modified based on real-time measurement results to improve overall data collection performance. A common example is when a real-time measurement result is used to optimize the preparation/dilution of a sub-sample matrix to match the dynamic range of either future real-time measurements, or for follow-on analysis of the same sample by a more definitive technique.
- A third example is when the over-all analytical strategy is changed in response to real-time results. This could be in response to the presence of previously unidentified contaminants of concern. Overly rigid standard operating procedures (SOPs) can sometimes obstruct the analytical flexibility required to modify or substitute methods (preparative or determinative) to accommodate complex environmental samples, or to handle unexpected contaminants of concern encountered in the field. Adaptive analytics selection strategies can be used to introduce the required flexibility. Adaptive analytical selection strategies are consistent with Performance Based Measurement System (PBMS) concepts.
- A fourth example is the use of focused analytical QA/QC protocols. Dynamic strategies in a focused QA/QC program refer to strategies that allow for rapid problem identification and correction as work is underway in response to real-time results. These QA/QC strategies can support fixed-laboratory analytical programs, as well as real-time measurement systems that are field-deployed. Focused QA/QC strategies can include:
- Front-end loading QC efforts to flush problems out earlier rather than later, eventually reducing the level of QC effort when comfort levels with technology performance are raised.
- Identifying samples with particular characteristics that make them of special interest from a QC perspective (e.g., samples below the cleanup level if false negatives are a concern, or just above if false positives are an issue).
- The use of method applicability studies to test and refine measurement system performance for decision needs, identify appropriate QA/QC requirements, and determine how best to use various data sets collaboratively to support decision requirements.
|
 |
 |