 |
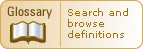
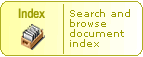
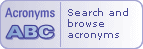
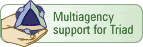
Triad is a Federal/State Interagency Partnership
|
 |
Identifying and Managing Uncertainty
The Triad emphasizes managing decision uncertainty through appropriate and cost-effective data collection that makes use of dynamic work strategies and real-time measurement technologies when possible.
The emphasis of the Triad is on managing decision uncertainty during the hazardous waste site characterization and remediation process. Several key concepts important to the discussion of uncertainty identification and management were introduced in the section entitled Overview and Key Concepts. Recall that within the context of the Triad approach, the term "uncertainty" is used broadly to refer to things that are unknown or incompletely understood. This section discusses uncertainty concepts important from a Triad project management perspective in more detail.
There are plenty of examples of what happens when decision uncertainty is not properly managed within the hazardous waste site characterization and remediation process. Common cases include:
- "Closure" achieved for sites, with subsequent contamination discovered that requires the site to be reopened.
- Groundwater treatment systems that cost hundreds of thousands of dollars to install and operated at an additional cost of tens of thousands of dollars per year, yet cleanup does not appear to be making progress.
- Ad hoc, expensive characterization programs that do not resolve fundamental site questions, requiring remobilization for additional data collection.
- Nature and extent of soil or sediment contamination whose characterization was incomplete or inaccurate, leading to additional remedial activities with significant cost and schedule overruns.
- Brownfields redevelopment that is hobbled by the uncertainty associated with potential environmental liabilities and their financial risks.
Sources of Uncertainty
The basic sources of uncertainty associated with hazardous waste sites include:
- Decision Uncertainty. Decision uncertainty is equivalent to the likelihood of making the wrong decision, such as concluding that an area complies with cleanup criteria when in fact it does not. Decision uncertainty is the opposite of decision confidence. Decision quality is the degree to which an actual decision coincides with the decision that would have been made if complete and fully accurate information was available at the time. Cost-effectively managing decision uncertainty is the primary focus of the Triad for hazardous waste site cleanup activities. Decision uncertainty is the aggregated uncertainty contributed by political, economic, and public perception factors, along with model, analytical, sampling, and relational uncertainties. The last three, taken together, are often referred to as data uncertainty.
- Model Uncertainty. Model uncertainty refers to the uncertainty associated with the "correctness" of models (e.g., fate and transport, risk pathway analysis, etc.) used in a CSM to represent key features or characteristics of a hazardous waste site. Correctness refers to the ability of a model to accurately portray a key site feature (e.g., risk pathway, fate and transport prediction, etc.). Key site features are those important for decision-making purposes. Model uncertainty is distinct from the uncertainty introduced into a CSM, its associated models, and decision-making by data collection efforts. Uncertainties associated with data include analytical, sampling, and relational uncertainties. Models make use of data sets, and can amplify data uncertainty when making decisions if significant model uncertainty also exists.
- Analytical Uncertainty. Analytical uncertainty refers to the uncertainty associated with analytical results for media samples. Analytical uncertainty stems from the limitations of analytical and determination methods. Common contributors to analytical uncertainty include poor detection limits, analytical bias, lack of precision, and susceptibility to interferences. Analytical uncertainty and its reduction have been the primary focus of uncertainty management for traditional cleanup programs, but the contribution of analytical uncertainty to overall decision uncertainty is often insignificant compared to other sources of uncertainty (e.g., sampling uncertainty).
- Sampling Uncertainty. Sampling uncertainty reflects the degree to which sample results represent actual conditions for the population sampled, neglecting the contributions of analytical or relational uncertainty. Sampling uncertainty is an inclusive, catch-all phrase referring to all factors not associated with the analytical method that contribute to data uncertainty. The primary contributors to sampling uncertainty are the natural heterogeneity in contamination distributions across the area being sampled and the potential non-representativeness of sampling procedures. Other contributors include problems with sample processing (e.g., improper handling, incomplete sampling equipment decontamination, etc.). Sampling uncertainty's contribution to data uncertainty is often significantly greater than that from analytical uncertainty.
- Relational Uncertainty. Relational uncertainty is the uncertainty associated with the relationship between a parameter being measured, and the true parameter of interest from a decision-making perspective. Relational uncertainty can become a concern for real-time measurement methods that are non-specific in nature (e.g., immunoassay kits), or that measure a parameter other than the primary parameter of concern using the measured parameter as a proxy for the primary parameter when site decisions are made. Relational uncertainty can be of concern for traditional data collection programs when traditional fixed-laboratory methods produce data used as a surrogate for more difficult to obtain information. For example, total chromium concentrations are sometimes used as a proxy for the toxic chromium(VI) fraction, and total metals concentrations are often used to estimate exposure risk if estimates of the bioavailable fraction are not available.
Managing Uncertainty
Project managers must understand a few basic facts about hazardous waste site decision uncertainty and its management:
- Decision uncertainty is a fact of life for environmental decision-making. It is unavoidable.
- Completely eliminating decision uncertainty (and the possibility of decision errors) is impossible. The best one can accomplish is to manage uncertainty in a manner that keeps the possibility of making mistakes at acceptable levels.
- There are diminishing returns to investments in reducing decision uncertainty. Sampling uncertainty provides a good example. In general, sampling uncertainty is reduced as the square root of additional sample numbers. To reduce sampling uncertainty by a factor of four often requires increasing sample numbers by a factor of sixteen.
Managing decision-making uncertainty presumes that one can identify and prioritize the primary contributors to decision-making uncertainty. Political, economic, and social factors may be important sources of uncertainty for site decision-making (e.g., cleanup standards changing with time, or funding constraints imposed by state or federal agencies impacting decisions already made). It is important that these contributors to decision uncertainty be identified and their potential implications understood to the degree possible as part of the systematic planning process, but resolving these issues is beyond the scope of the Triad.
Directly addressing numerical or analytical model uncertainty is also beyond the scope of the Triad. Method development for identifying and quantifying model uncertainty is an active area of research (e.g., Monte Carlo and stochastic simulation methodologies applied to fate and transport modeling or risk estimation). Project managers must recognize that this source of uncertainty can be significant, if not completely quantifiable. Predictive fate and transport model results are often presented as definitive, when in fact the physical systems governing fate and transport may be poorly understood and incorrectly modeled. There are many historical examples of contamination plumes developing in a manner that was not predicted by models, or responding to remedial activities in ways that were not foreseen (e.g., contaminated groundwater going around a reactive subsurface barrier). The Triad can contribute, however, to the development of dynamic work strategies that address the uncertainty introduced into decision-making by model uncertainty. An adaptive site remediation strategy is one such option that will be discussed in greater detail in the section entitled Dynamic Work Strategies.
The Triad's main focus is on managing decision-making uncertainty by carefully considering all possible options to achieve desired project outcomes. Where the decision process requires developing a more complete CSM through the collection of environmental data, the Triad focuses on managing data uncertainties associated with the data collection process. The increased costs this would incur under conventional work strategies is more than offset by the Triad's reliance on dynamic work strategies to refine the CSM and guide characterization or remedial activities in real-time.
All environmental projects rely on data collection to provide inputs into the decision process. While not every site has a complex fate and transport model, all hazardous waste sites will at some stage make use of data collection to further refine a CSM and support a decision that must be made. Based on past experience, for traditional fixed-laboratory-based sampling and analysis programs, the primary contributor to data uncertainty is sampling uncertainty. Consequently, the Triad has centered on cost-effective methods to reduce sampling uncertainty. Another way of expressing this is that the Triad is committed to achieving acceptable levels of decision uncertainty at least cost, and the primary vehicle for reaching that goal is by addressing the sampling uncertainties in the data generation process.
For many project decisions, good solid common sense and proactive project planning will be all that is necessary to address uncertainty associated with a decision. Examples include decisions where the possible results of a "wrong" choice are inconsequential, or the decision options are constrained to the point that there is only one path forward, or the cost of reducing uncertainty is prohibitive compared to the implications of an incorrect decision. In these cases, uncertainty management may simply consist of documenting the basis for the decision, identifying potential project outcomes that are possible but not expected in the context of the decision made, and providing for contingency plans to handle those outcomes if they occur.
When a review of decision uncertainty indicates that there are key information deficiencies contributing to uncertainty, and that those deficiencies are significant enough to justify the cost of additional data collection, then the two other legs of the Triad, dynamic work strategies and real-time measurement systems, become important tools for efficient decision uncertainty management. These two Triad legs are discussed in greater detail in the sections entitled Dynamic Work Strategies and Real-Time Measurement Systems. For the purposes of this section, however, there are several salient points project managers should remember.
- The most effective uncertainty management strategy often relies on a weight-of-evidence approach to decision support. A weight-of-evidence approach recognizes that there may be several sources of information contributing to a decision that must be made, and that those sources cannot always be combined in a quantitative way. For example, to support a no further action conclusion during a CERCLA PA/SI or to satisfy due diligence for a property transaction, historical records, interviews, site walk down observations, historical aerial photographs, process knowledge, and limited judgmental sampling results may all contribute to the final conclusion.
- Within a weight-of-evidence approach, sources of information other than physical samples may prove much more effective and cost-efficient at establishing an appropriate "comfort level" with key decisions than media sampling. For example, if the concern is the potential for buried drums, a non-intrusive geophysical survey will more likely determine the presence or absence of concerns than any affordable amount of subsurface soil sampling.
- Where data collection in the form of physical sampling is warranted, judgmental samples can be extremely effective if a CSM is in place that adequately justifies location selection and establishes how the results from samples will be used to validate/invalidate the CSM and thus support decision-making. In this setting, judgmental samples are typically used to establish the presence or absence of some key parameter at "worst case" locations (e.g., the presence or absence of contamination at an outfall pipe, or associated with a subsurface anomaly identified by a non-intrusive geophysical survey), or the presence or absence of a contamination event in some general area.
- For data collection programs with limited sample numbers, sampling uncertainty usually outweighs analytical uncertainty, and often by wide margins. Sampling uncertainty is primarily addressed by increasing sample numbers and controlling for variables such as sample support. Analytical uncertainty is primarily addressed by appropriate QA/QC procedures and fine tuning analytical methods to meet site specific needs. The proper balance between investments in reducing analytical uncertainty versus investments in reducing sampling uncertainty can be determined by a cost-benefit analysis. Both investments, in turn, need to be balanced with the cost of making a wrong decision. The level of analysis should be proportional to the stakes, e.g. if data collection will consume significant resources and/or the implications of wrong decisions are significant, then relatively more should be spent on getting the right balance. A simple rule of thumb: if the per unit cost of remediating soil is insignificant, then there may be little reason to invest in either reducing sampling or analytical uncertainty. Decision uncertainty in this case would be managed by over-remediation. As per unit remediation costs increase, the value of addressing sampling and/or analytical uncertainty increases as well.
- Significant cost and schedule efficiencies can be gained if sampling programs are structured in a manner that prioritizes activities based on maximizing uncertainty reduction. The point is that in some cases the conclusion to a decision statement becomes obvious after only a few well-placed samples if their results are immediately available, making subsequent data collection unnecessary. As a simple example, suppose the goal of sampling work was to support a NFA conclusion for a particular parcel of land, and judgmental sample collection targeted locations identified by the CSM as having the greatest potential for harboring contamination. An adaptive approach to field work would proceed from the most likely contamination location to the least likely. If contamination was encountered at any particular location at levels that would prevent a NFA from being achieved, sampling could stop or be redirected for the purpose of delineating what was discovered.
Significant cost saving and decision quality improvements can also be obtained if an adaptive approach is applied to remedial designs as well. This can be a particularly effective approach for managing decision and CSM uncertainty introduced by sparse historical data or by model uncertainty. For example, real-time data collection might be integrated with excavation procedures so that uncertainty about the contamination status of subsurface soils is resolved by exposed surface data collection while excavation is underway. Options for how to do this are discussed in more detail in the section entitled Dynamic Work Strategies.
Uncertainty Management Table Examples
Navy Developed Data Uncertainty Calculator
|
 |
 |