 |
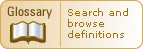
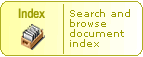
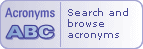
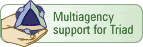
Triad is a Federal/State Interagency Partnership
|
 |
Analytical Performance Parameters
Parameters used to characterize the performance and quality of analytical methods in Triad projects.
There are a number of parameters used to characterize the performance and quality of analytical methods. These are discussed briefly below:
- Detection/Quantification Capabilities. The detection capability is the lowest analyte amount that can be expected to be detected at a given confidence. Often there is high uncertainty associated with reported quantitative results near the detection limit. Numerical results should be expected to be unreliable when reported near the detection capability of a method. This is not a problem with the laboratory; it is the nature of the science of analytical chemistry. In contrast, the quantification capability is the lowest amount of analyte that can be measured and reported as a quantitative result with a given amount of statistical confidence. Different organizations have different rules for how laboratories are expected to determine these various levels and what level of statistical confidence is chosen for each. Different permutations often have different names, adding to the confusion.
Data users should be aware that detection/quantification concepts, although apparently straightforward, are anything but simple in real-world analytical systems. This is particularly true for environmental analysis of pollutant trace levels (ppm concentrations and lower). The detection and quantification limits estimated by laboratories are often generated using highly idealized analytical scenarios and matrices. Reported detection and quantification limits can be misleading when limits estimated from idealized systems are extrapolated to real-world matrices and analytical systems without compensating for real-world deviations from the ideal. This can lead to both false positive and false negative detections, resulting in decision errors. Data users may also attach much more confidence than is warranted to a single numerical result, falsely believing that a result reported as "5.5" means exactly 5.5, whereas in actuality that result is only a point estimate for a value that statistically could lie anywhere between 3 and 8.
- Selectivity/Specificity. Selectivity is the ability of a particular analysis method or technology to identify and quantify a particular compound in the presence of other, potentially interfering compounds. The word "interference" is usually reserved for non-target or unrelated analytes or substances that falsely increase or decrease or obscure the response of the target analyte(s). All methods, including traditional fixed laboratory methods, are subject to interferences that can render results unreliable unless corrective actions are taken. Those actions include things like using cleanup methods prior to analysis to remove the interfering substances (in the case of some organic analyses), or using wavelength scans to detect and correct for spectral interferences (in the case of some metals analyses).
The term "specificity" is used to express the same general idea as "selectivity," but to an even higher degree. A test with a high degree of specificity will not only resist non-target interferences, but also give unique responses that permit closely related compounds to be distinguished from a unique target analyte. Non-specific methods are designed to give a response that integrates the individual responses of all responding target analytes present in the sample. This is common, for example, with immunoassay test kits, where the term "cross-reactivity" is used to describe the integration of all responding target analytes into a single summed response.
Selectivity and specificity are important concepts for the Triad because several field-deployable real-time measurement options measure the presence of a compound class. An immunoassay test kit may be reasonably selective for a certain class of compounds, such as the cyclodiene class of chlorinated pesticides. However, the response of an immunoassay cyclodiene test kit may not distinguish between the twelve or more specific compounds within the cyclodiene class. Because the test kit is non-specific, all compounds in that class are target analytes from the kit's viewpoint. However, not all of the kit's target analytes will be of equal regulatory concern. A test result that is less than the decision threshold has a high predictive value for a regulator because it indicates that no compound in that group exceeds the threshold determined to be of regulatory interest. But a result greater than the threshold indicates that at least one compound in the class might be present at levels of potential concern. Unless prior knowledge exists about what pesticides are present in the sample, more analytical work would be required to know exactly which compounds are present and in what concentration.
Designing data collection and interpretation protocols using non-specific methods requires technical expertise to understand what the method is measuring in relation to how the data will be used for decision-making purposes. Quality control procedures (such as performance evaluation samples) must also be adapted to correctly match the method's characteristics. Complaints that "field methods don't work" sometime stem from incorrect interpretation and misuse of results from non-specific methods. But in knowledgeable hands, these techniques can provide reliable, quality-assured data supporting highly confident decisions.
- Precision. Precision is a measure of the random error or variability observed in measurement results that is a product of the sample handling and analytical process. Precision is typically expressed as the standard deviation found in sample results. For environmental data sets, it is important to separate variability that is the product of spatial heterogeneity in contaminant distribution, and random variability that is introduced by the sampling/analysis process. The former is not connected to measurement precision.
- Bias. Bias refers to systematic differences between measurement results and the true value of a parameter one is measuring. Bias in measurement results can be introduced in a number of ways, including through sampling, sample handling, sample preparation, matrix interference, cleanup and determinative processes. The term "accuracy" is sometimes used to capture the combined effects of precision and bias. Certain methods may be known to be biased. For example, most immunoassay test kits manufactured for environmental use in the U.S. are deliberately designed with upwards of 100% positive bias. This will have ramifications for the design of quality control procedures, such as comparing performance evaluation (PE) control results with reference values. The degree of bias (if it is present) should be documented by the project-specific method QC. Understanding whether bias exists and its impact on decision-making is important to demonstrate that data are of known and documented quality. Whether (and how) bias is compensated for in the decision-making process should also be documented in project reports.
- Comparability. The EPA Office of Environmental Information Quality defines comparability as: "A measure of the confidence with which one data set or method can be compared to another." [USEPA QA/G-5] Comparability is an extremely important concept for the Triad approach because typically more than one analytical method will be used to analyze for the same analytes. This concept is discussed in greater detail in a following section.
- Representativeness. The USEPA Office of Environmental Information Quality defines representativeness as: "A measure of the degree to which data accurately and precisely represent characteristics of a population, parameter variations at a sampling point, a process condition, or an environmental condition. Representativeness is also the correspondence between the analytical result and the actual environmental quality or condition experienced by a contaminant receptor." [USEPA QA/G-5S]
Environmental sampling collects small samples from which even smaller portions may be analyzed with the idea that the results from tiny samples will be extrapolated back to the tons of soil or water from whence the sample came. In other words, the analytical results from tiny samples are expected to "represent" the analyte concentrations for the original bulk matrix that was sampled. The problem with this simple concept is that environmental matrices are extremely heterogeneous: the results from two soil samples inches apart can be completely different. Concentrations of contaminants in groundwater in a single well have been shown to vary by several orders of magnitude within several feet of vertical depth. In the face of heterogeneity, how can the idea of representativeness be made useful?
Like other Triad concepts, Triad grounds the concept of data representativeness in the decision-making process. Data from heterogeneous matrices must be collected in a way that is representative of the exact decision being made. In other words, the data generation process must sample the environment in a way that mirrors the decision. Even for the same bulk matrix, different risk and engineering decisions may require different data representativeness when contaminants are distributed heterogeneously. For example, consider a decision determining exposure risk to lead-contaminated soils blowing off-site into residential homes where the dust may stick to children's fingers and become ingested. Previous studies have documented that soil lead concentrations can be inversely correlated with particle size. The smallest particle sizes (less than 200-mesh or 0.07 mm) can have lead concentrations as much as 200 times higher than lead concentrations for larger particle sizes (about 1 cm). If site soil is sampled to give an "average" concentration for the bulk soil without accounting for the particle size that represents the mechanism of lead exposure, then the soil data may seriously underestimate actual exposure concentrations.
Systematic planning determines the sampling and analytical procedures that will produce data that are explicitly matched (i.e., representative) to the decision. The more heterogeneous the matrix composition and contaminant distribution are, the more carefully project planners must consider all of the sampling and analytical variables that contribute to data variability. It is crucial to use a CSM that predicts and tests assumptions about heterogeneity and sampling variability.
The performance of a particular analytical method is not a pre-determined constant. As already described, there are a number of site and project-specific parameters that will affect the analytical quality of data produced by any particular technique. For most methods, analytical quality can be improved (i.e., analytical uncertainty reduced) with sufficient resource investments.
|
 |
 |