 |
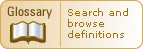
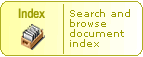
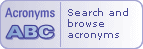
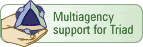
Triad is a Federal/State Interagency Partnership
|
 |
Analytical, Data, and Decision Quality
Cost-effective approaches to efficiently manage uncertainties introduced by the complete data quality chain, including sampling, analytical, and relational uncertainties.
Traditional approaches to hazardous waste site data collection design are based on a first-generation data quality model. Under this paradigm, decision quality is synonymous with analytical quality. The result was an emphasis on expensive standardized analytical techniques from fixed laboratories that provided data of the highest available analytical quality. Experience, however, has demonstrated that for many sites the leading contributor to decision uncertainty has been sampling uncertainty caused by limited sample numbers or samples not adequately representative of the matrix of concern, not analytical uncertainty.
The Triad uses a second-generation data quality model to address decision uncertainty and decision quality. This second-generation model is based on two fundamental principles: (1) Environmental matrices should be assumed heterogeneous in composition and contaminant distribution, unless shown to be otherwise and (2) "data quality" is assessed according to the ability of data to provide the data user with accurate information to support correct decisions. In the context of this second-generation data quality model, the Triad uses cost-effective approaches to efficiently manage all of the uncertainties introduced by the complete data quality chain, including sampling, analytical, and relational uncertainties.
The concept of collaborative data sets is important to the Triad. Collaborative data sets refer to data that have been generated by more than one technique, and that, when taken together, provide what is necessary to confidently make decisions. An example of a collaborative data set would be a data set with relatively few, high analytical quality results that are used to manage analytical error, and comparatively greater numbers of cheaper, lower analytical quality analyses that address sampling uncertainty and support development of a confident contaminant distribution model (i.e., a CSM). Of course, the ideal situation would be the ability to field a data collection program that inexpensively and exhaustively characterized a site with relatively definitive analytical results. With a few exceptions, however, this ideal is not achievable for most characterization and remediation programs. The challenge for most sites is to determine the optimal mix of data collection technologies and analytical methods that satisfy decision quality requirements at least cost.
An important concept related to decision quality is that of the "region of decision uncertainty" or "uncertain region." The uncertain region represents a result or set of results that cannot be used to make a decision with the level of confidence needed to satisfy decision-makers and stakeholders. The concept of an uncertain region can be applied to a single result (e.g., Does this value indicate that the sampled location is above cleanup levels?), to a set of results (e.g., Is the average sample result for an area below cleanup requirements?), or to a statistical test (e.g., Does the Student t test establish that the null hypothesis should be rejected at a 95% confidence level?).
An uncertain region example is the application of a field test kit to identify the presence of highly elevated areas or hot spots. Suppose the elevated area cleanup requirement is 50 ppm. Experience with the test kit demonstrated that as long as the kit measured less than 45 ppm, a traditional fixed-laboratory analysis would not likely yield a result greater than 50 ppm. On the other hand, a test kit result greater than 125 ppm almost always corresponded to a fixed-laboratory result greater than 50 ppm. In this example, test kit values below 45 ppm or above 125 ppm would be considered sufficient for decision-making purposes, but the range between 45 and 125 ppm would represent the uncertain region. The size of the uncertain region in this example could potentially be reduced by addressing analytical quality (e.g., improving sample preparation and cleanup procedures, switching to an analytical method with intrinsically better analytical quality, increasing the number of specific types of QC samples, etc.).
In many instances, standard fixed-laboratory results are treated by decision-makers as though they are completely definitive, or in other words that there is no uncertain region associated with analytical results for decision-making purposes. This is a decision-making simplification that does not reflect the reality of analytical science or the nature of how pollutants behave in the environment. At best it provides a false sense of certainty with regard to decision quality, and at worst can produce incorrect decisions, or lead to disagreement and decision-making confusion when duplicate analyses and/or sample splits analyzed by another laboratory or alternative techniques are not consistent in their results.
Statistical analyses of data sets provide another example of uncertain regions. Suppose, for example, there is a requirement that the 95% upper confidence limit (UCL) on the mean value for a set of samples must be less than the cleanup requirement. In this case, there would be a range of computed average values that are less than the cleanup requirement, but not low enough to bring the 95% UCL below the requirement. This range represents an uncertain region for decision-making purposes. One could not conclude with the desired statistical confidence that the true population mean met the requirement. The size of the uncertain region in this example is controlled by the number of samples contributing to the mean, the confidence level required, and the level of contamination concentration heterogeneity present. The size of the uncertain region could be reduced by increasing sample numbers or relaxing confidence level requirements.
The important points in both examples are that the uncertain region is always present, but that its size (and consequently its impact on decision-making) can be controlled by proper selection and implementation of analytical methods and sampling protocols. The uncertain region's size is also a function of the level of certainty required to support decision-making. For example, if one insisted on very small decision-making error rates the uncertain region's size would be much larger than if one was relatively tolerant of incorrect decisions.
The uncertain region's impact on decision-making is affected by the actual spatial concentration distribution of contamination. In the test kit example, if a site either was un-impacted or consistently impacted at levels well above 55 ppm, then the effectiveness of the test kit for making definitive project decisions would be excellent. If, on the other hand, there were large portions of the site where true concentrations were around 50 ppm, then one would expect that the kit results would have much less predictive power from a decision-making perspective for those areas. Understanding the interactions between analytical quality, sampling uncertainty, cleanup level definitions, and contaminant distribution is key to designing an efficient sampling program and selecting the right mix of analytical technology options for a Triad project.
A concept related to uncertain regions is that of field-based action levels. Field-based action levels are also sometimes referred to as investigation levels or trigger levels. Field-based action levels are decision levels used during field activities to support decision-making based on real-time results. For example, on-site results may be compared to a field-based action level for the purpose of deciding whether a waste or matrix volume is contaminated above requirements, or should be relegated to one disposal option versus another. An uncertain region's upper and lower bounds are a natural choice for field-based action levels since these identify break-points in analytical results that can be used to support confident decision-making. There are a number of methods for determining technology and/or decision-specific uncertainty regions and field-based action levels, including parametric and non-parametric statistical techniques.
A final important data quality fact is that the analytical quality for a particular technique is not a fixed quantity, but is rather dependent on several factors. These include the particular analyte of concern for those methods (such as GC-MS or ICP) that are capable of returning results for multiple elements or compounds, the presence or absence of interferences in the sample matrix, the level of effort invested in sample preparation and cleanup, the amount of QA/QC imposed on the technique, the degree of control over environmental factors such as heat and humidity, and the skill and experience of the analyst. Given these facts, it is important to recognize that the analytical quality produced by a generic method may range from acceptable to unacceptable depending on analyte-, site-, and project-specific factors. In general, improving analytical quality for a specific method (whether field or fixed laboratory-based) increases per sample costs and decreases sample throughput rates. Consequently, there is a trade-off between managing analytical uncertainty and managing sampling uncertainty for data collection programs that rely on one analytical method and that operate on a fixed budget. Increasing analytical quality comes at the expense of decreasing the number of samples that can be afforded. From a Triad perspective, the proper balance is reached when decision uncertainty is minimized.
|
 |
 |